February 2020: Yuhao Zhang
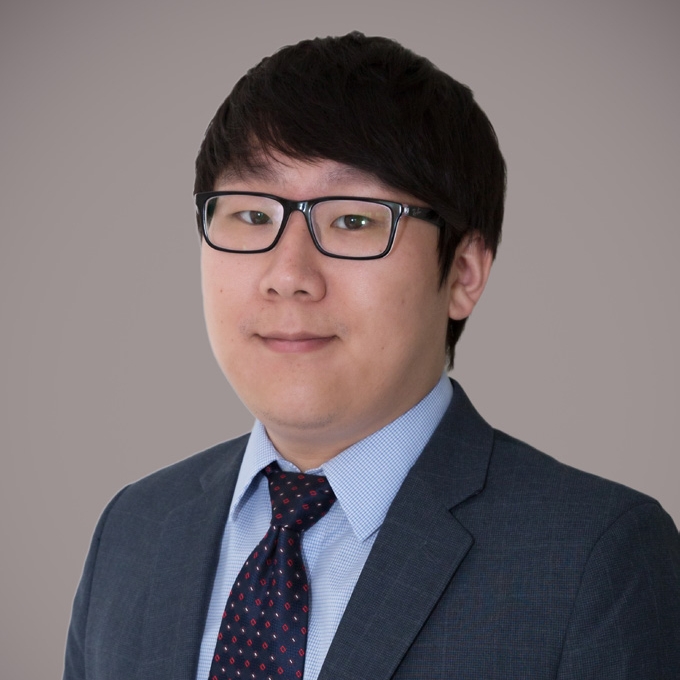
Yuhao Zhang
Assistant Professor
Department of Electrical and Computer Engineering
PhD, Electrical Engineering, Massachusetts Institute of Technology, USA
MS, Electrical Engineering, Massachusetts Institute of Technology, USA
BS, Physics, Peking University, China
Email yhzhang@vt.edu
How do you see your work contributing to the goals and vision of IIHCC?
Essentially, one of the main objectives of my work is to develop a new generation of materials, the (ultra-)wide-bandgap semiconductors, and the related devices that can enable system-level benefits to all intelligent systems; not only power systems but many others like internet of things (IoT), solar energy systems, smart buildings/architecture, etc. Our first goal is to develop new materials and devices to be used for energy conversion and to make energy conversion more efficient. The second goal is to simplify system-level design, which can reduce the form factor of the system—size, weight, and volume. Ultimately, if you combine these two goals together, then we can envision lower costs for all these systems. Finally, the device or the materials that we develop are not only more efficient for energy processing but can also enable higher efficiency in energy harvesting, which will be important for wireless connection because the wireless connection is the foundation for IoT. The overall energy efficiency of wireless connection is still very low, and this is one of the limitations because a lot of the energy is lost during transformation. Basically, our devices and materials contribute to ensure that energy flow, energy processing, and energy communi-cation occur with high efficiency, bringing a great amount of benefits to all the applications of the system. Top- level applications may benefit from the circuit to the system, from consumer electronic products to very large-scale systems like the Grid and buildings.
What other areas outside of your discipline would you entertain for future research and proposal work?
I am trying to see the interaction of fundamental system processing with machine learning and artificial intelligence. For example, in semiconductor processing, typically devices are manufactured on a wafer. One of the key challenges for the semiconductor industry is how to improve the yield of wafer production. The prerequisite to improve the yield is to categorize every device in a wafer, understand if the performance is outside the range of our expectations, and determine the root cause for the variance. This variance could be from one of many manufacturing steps or could be due to the nonuniformity of the materials or the wafer itself. As you can imagine, it can be very time consuming and costly, and it is not easy to go through every wafer and identify what is the root cause for the variation. That is why for any generation of devices, hundreds of billions of dollars have been spent on these processes. So, I want to research how machine learning can play its role in process variability analysis. If we can first identify the roots causes for poten-tial variations in device performance, then we can train the machine learning algorithm using the collected data. If that algorithm is trained very well, then, for any device performance that it measures, the algorithm can tell you the root cause of this variation.
One field I am currently exploring is the application of machine learning in microelectronics and power electronics. For example, you have potential variation that may appear in device design, circuit design, etc. because these designs are essentially based on the experiences of the designer. We can apply machine learning to these designs because it can accommodate in the processing of more components and consider more combinations compared to any designer’s existing experience. I think this can potentially lead us to achieve the global optimization for hardware systems that involve material-device-circuit inter-plays.